Artificial Intelligence has rapidly become an integral part of our daily lives, influencing decisions in healthcare, finance, hiring – and beyond. While AI promises efficiency and objectivity, it also carries the risk of perpetuating and amplifying existing societal biases. Understanding the extent of bias in AI and the measures being implemented to address it is crucial for building fair and equitable systems.
Understanding AI Bias
AI bias refers to systematic and repeatable errors in a computer system that create unfair outcomes, such as privileging one arbitrary group of users over others. These biases often stem from the data used to train AI models, which may reflect historical inequalities or prejudiced human decisions. For instance, if a hiring algorithm is trained on data where certain demographics were historically underrepresented, it may learn to favour candidates from overrepresented groups, thereby perpetuating existing disparities. arXiv
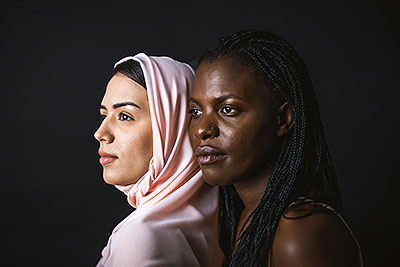
Real-World Implications
The consequences of AI bias are not merely theoretical; they have tangible impacts on individuals and communities.
Healthcare Disparities: AI systems used in medical diagnostics have shown varying accuracy across different demographic groups. For example, algorithms trained predominantly on images of lighter-skinned individuals may underperform when diagnosing conditions in patients with darker skin tones, leading to misdiagnoses and delayed treatments. magazine.publichealth.jhu.edu healthcare-in-europe.com
Financial Inequities: Credit scoring algorithms may inadvertently disadvantage minority applicants if they rely on data that reflects historical lending biases. This can result in unfair denial of loans or higher interest rates for certain groups. BABL AI
Employment Discrimination: AI-driven recruitment tools have been found to favour candidates based on patterns in historical hiring data, which may exclude qualified individuals from underrepresented backgrounds.
Efforts to Mitigate Bias in AI
Recognising the challenges posed by bias in AI, researchers, policymakers, and organisations are actively working on solutions to create more equitable AI systems.
1. Regulatory Frameworks
The European Union has taken significant steps to address AI bias through legislation. The EU’s Artificial Intelligence Act aims to ensure that AI systems are developed and used in ways that respect fundamental rights. Article 10 of the Act emphasises the importance of high-quality datasets, mandating that training, validation, and testing data be relevant, representative, and free from errors. This provision seeks to minimise biases that could lead to discriminatory outcomes. The Sun EU Artificial Intelligence Act
2. Bias Mitigation Techniques
Technical strategies are being employed to reduce bias in AI models: eLearning Industry
Pre-processing: Modifying training data to eliminate biases before feeding it into the model.
In-processing: Incorporating fairness constraints into the model’s learning algorithm. uxmatters.com
Post-processing: Adjusting the model’s outputs to correct biased results. uxmatters.com
These methods aim to create models that perform equitably across different demographic groups.
3. Inclusive Data Collection
Ensuring that AI systems are trained on diverse and representative datasets is crucial. Initiatives like the SHADES dataset focus on evaluating and mitigating AI bias across multiple languages and cultures, acknowledging that biases can be more pronounced in non-English contexts. WIRED
4. Advocacy and Awareness
Organisations such as the Algorithmic Justice League, founded by Joy Buolamwini, work to highlight and combat AI bias. Through research, policy advocacy, and public engagement, they aim to promote accountability and transparency in AI systems. Wikipedia
Challenges Ahead
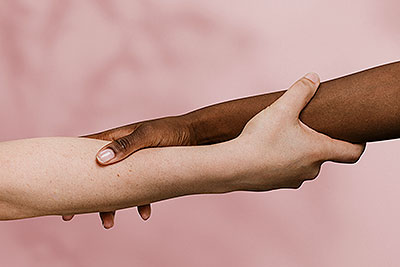
Despite these efforts, significant challenges remain:
Complexity of Bias: Biases can be subtle and multifaceted, making them difficult to identify and rectify.
Dynamic Societal Norms: What is considered fair or biased can evolve over time, requiring continuous monitoring and adaptation of AI systems. arXiv
Global Deployment: AI systems deployed globally must account for diverse cultural contexts, which adds complexity to bias mitigation efforts. WIRED
Final Thought
AI bias is a significant concern with real-world implications, but it is not insurmountable. Through a combination of robust regulatory frameworks, technical interventions, inclusive data practices, and active advocacy, strides are being made to create fairer AI systems. Continued collaboration among stakeholders is essential to ensure that AI serves as a tool for equity rather than a perpetuator of existing disparities.
Victor A. Lausas
Chief Executive Officer